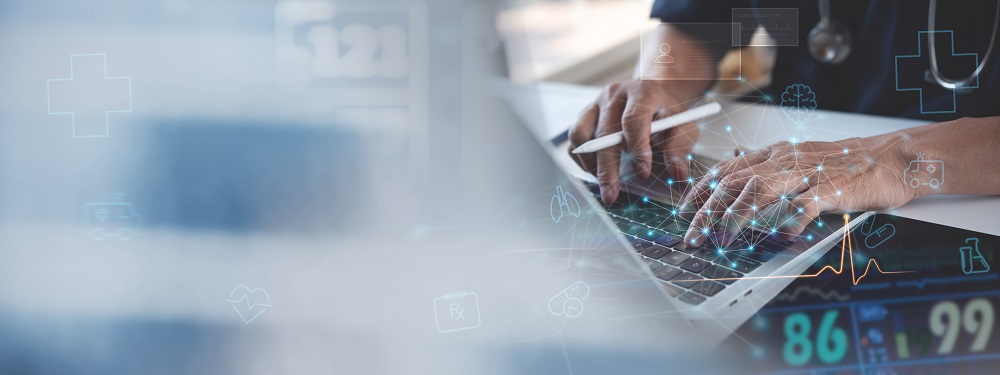
Though using large language models (LLM) that drive artificial intelligence (AI) to draft patient notes show promise in saving physicians time, they will still need to review and edit these summaries for accuracy, according to researchers and colleagues at Weill Cornell Medicine. Their findings suggest that as the LLM system is refined to handle documentation more accurately, physicians will have more time to focus on patient care, if they only need to review AI-generated notes versus manually producing them. This may also address concerns about physician burnout due to time-consuming administrative tasks.
Dr. Rahul Sharma, Chair of the Department of Emergency Medicine, Founder of the Center for Virtual Care at Weill Cornell Medicine, and co-author on the study, has been leading the development of innovative programs in virtual care and digital health as well as physician wellness before the pandemic made these issues front of mind. Most recently, the department was recognized by the American College of Emergency Physicians with the Emergency Medicine Wellness Center of Excellence Award.
“The early results of this LLM study suggest that there are opportunities to improve care transitions and their related communications. Also, having more efficient and effective processes can reduce the burden on clinicians, thereby promoting wellness and alleviating burnout,” said Dr. Sharma.
In the study, published Dec. 3 in JAMA Network Open, the researchers evaluated a customized LLM which generated handoff notes based on de-identified emergency room admissions records from 1,600 patients at NewYork-Presbyterian/Weill Cornell Medical Center in 2023. Such handoff notes or summaries are sent to inpatient teams who are taking over the care of patients. The LLM’s automated notes initially looked superior to the manual doctor’s notes, but when further examined by physicians, they found inaccuracies in how the LLM interpreted and prioritized data.
“Before you implement a large language model into clinical care, there needs to be human validation of the model's output, rather than just relying on automated evaluations,” said Dr. Peter Steel, associate professor of clinical emergency medicine at Weill Cornell Medicine and an emergency medicine physician at NewYork-Presbyterian/Weill Cornell Medical Center. “A qualified individual evaluating the AI notes is able to perceive gaps in performance based on their knowledge and experience which a machine cannot.”
Vince Hartman is CEO of Abstractive Health, a Cornell Tech AI startup that developed the preliminary LLM model used in the study. An expert commentary also discussed the promising findings.
Handoff Notes—Crucial but Laborious Part of Patient Care
Handoff notes, an important part of health care, summarize a patient’s medical status, including key details about their diagnosis, treatment plan, recent changes in condition and any critical information that needs to be communicated when transferring care to another physician. For emergency medicine teams, creating manual handoff notes for the inpatient hospital staff takes time and each situation has unique circumstances creating opportunity for risk, as identified in prior research.
“Automating the process may make it more likely for physicians to have handoff notes on every patient being transitioned from emergency care to inpatient hospital care,” said Hartman. “It could also be done immediately as the patient is being transferred.”
While the healthcare industry is looking at ways to adopt LLM models within patient care, it's mindful that AI can create hallucinations. These errors or factually incorrect information have the potential to impact patient safety.
Automating the Process with Accuracy
To avoid inaccuracies that an LLM may generate, the researchers worked with Abstractive Health to design a customized LLM for the study, using tens of thousands of emergency department medical records to train the system. The researchers also developed a framework, borrowed from the World Health Organization’s Patient safety guidelines, to evaluate the effectiveness of the LLM-generated summaries.
Three board-certified emergency medicine physicians trained in quality and patient safety reviews used the framework to compare a subset of 50 notes drafted by the LLM against those written by doctors. They looked at reliability, accuracy, usefulness, completeness and flagged anything that could potentially affect patient safety.
“We wanted to see not just how frequently the LLM results had hallucinations, knowledge gaps, faulty logic or biases, but whether those inaccuracies would likely impact patient safety ” Dr. Steel said.
When AI tools that measure text similarity evaluated the LLM-generated notes, the notes were found to be more detailed and closer to the original emergency department documents than manual notes by doctors. However, the physician reviewers found that the LLM notes produced slightly more errors than manual notes, although without any life-threatening patient risks.
Next Steps
Dr. Sharma and the research team believe future research will develop LLMs that generate more challenging medical notes, such as discharge summaries, created when a patient goes home. These need to include relevant details across an entire hospital stay that could be days, weeks or more. Further, they hope other researchers will consider adopting the study’s unique patient safety framework when evaluating LLM-drafted notes. Eventually less labor-intensive solutions will be developed to support implementation at scale, including the possibility of LLMs evaluating LLMs.
This study demonstrates the potential of LLM-generated handoff notes with physician oversight to create a new standard of care in emergency medicine. “While this study certainly supports the transformative potential for AI in streamlining safe and efficient care transitions from the Emergency Department to Inpatient settings, we must employ careful monitoring and robust evaluation to ensure these processes are truly optimizing and elevating patient care,” said Dr. Sharma.